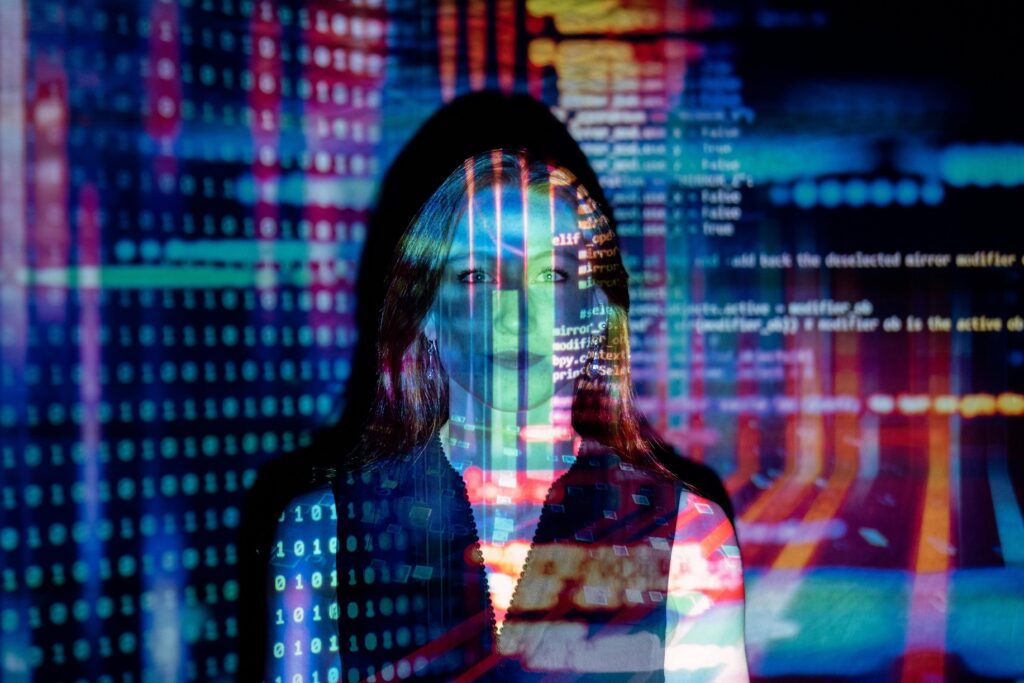
The World Bank has released a report emphasizing the urgent need for effective AI governance as artificial intelligence becomes increasingly integral to global economies and societies. The rapid advancement of AI technologies and their widespread adoption across various sectors, including healthcare, finance, agriculture, and public administration, present both unprecedented opportunities and significant risks.
Ensuring that AI is developed and deployed in an ethical, transparent, and accountable manner requires robust governance frameworks that can keep pace with technological evolution.
The report explores the emerging landscape of AI governance, providing policymakers with an overview of key considerations, challenges, and global approaches to regulating AI. It examines the foundational elements necessary for thriving local AI ecosystems, such as reliable digital infrastructure, a stable power supply, supportive policies for digital development, and investment in local talent.
As countries navigate this complex landscape, the report highlights the need to encourage innovation while mitigating risks like bias, privacy violations, and lack of transparency, emphasizing sustainable growth and responsible AI governance.
Regulatory Approaches to AI Governance
The report outlines four key regulatory approaches to AI governance, each offering distinct advantages and challenges:
1. Industry Self-Governance
– Strengths: Can directly impact AI practices if integrated into business models and company cultures.
– Limitations: Non-binding; not appropriate for high-risk sectors like finance or healthcare.
2. Soft Law
– Strengths: Includes non-binding international agreements, national AI principles, and technical standards; provides adaptable frameworks that promote responsible innovation.
– Limitations: Focuses on high-level principles rather than binding rights and responsibilities.
3. Hard Law
– Strengths: Binding legal frameworks provide clear, enforceable guidelines ensuring AI stakeholders comply with established standards.
– Limitations: Risk of becoming outdated and resource-intensive to implement.
4. Regulatory Sandboxes
– Strengths: Controlled environments allow for real-world experimentation with AI technologies, supporting innovation without exposing the public to unchecked risks.
– Limitations: Resource-intensive and have limited scalability, making them less feasible for wide-scale governance across diverse sectors.
Key AI Governance Challenges and Considerations
AI systems are inherently complex and dynamic, with implications that touch on ethical, legal, and socio-economic aspects. Governing AI requires frameworks that promote responsible innovation and mitigation, ensuring that AI’s benefits are distributed equitably while minimizing potential harms. These frameworks must consider sector-specific issues and legacy concerns, particularly in areas like healthcare, finance, and public services, where AI harms can scale rapidly.
One critical challenge is bias and fairness. AI systems, if not properly governed, can perpetuate and amplify existing societal biases, leading to unfair outcomes. Governance mechanisms must detect and mitigate bias at every stage of AI development and deployment, addressing pre-existing societal inequalities to prevent AI from entrenching or exacerbating them.
Another key issue is privacy and security. AI’s reliance on vast datasets raises significant concerns about data privacy and security, particularly where sensitive personal information is involved. Robust data protection standards and privacy-preserving AI techniques are necessary to safeguard individual rights and maintain public trust in AI technologies.
Transparency and accountability are equally crucial. AI decisions must be explainable, and developers must be held accountable for the impacts of their systems. Clear standards for explainability, coupled with mechanisms for auditing and oversight, are vital to maintaining public trust, especially in high-stakes sectors like finance and government.
Lastly, sustainable growth depends on reliable digital infrastructure, adequate power supply, and a robust talent pipeline. For sectors like agriculture or public administration, where AI can significantly enhance service delivery and efficiency, these foundational elements are crucial. Policymakers must ensure that legacy infrastructure is updated to support sustainable and inclusive AI growth.
Key Takeaways
AI governance cannot rely on a single, universal approach, and no regulatory model works in isolation. The report stresses the importance of adopting a flexible, adaptable governance framework that evolves with technological advancements and societal changes. Key takeaways include:
– Adopting a Multi-Stakeholder Approach: Engage diverse stakeholders, including industry, civil society, and academia, to ensure AI governance frameworks are inclusive and comprehensive.
– Tailoring Regulatory Mechanisms: Assess the maturity of the AI ecosystem, existing legal landscapes, and available resources when determining appropriate regulatory mechanisms.
– Promoting International Collaboration: As AI technologies transcend borders, international cooperation is essential to harmonize standards, address cross-border challenges, and ensure AI aligns with global public goods and human rights.
– Sector-Specific Considerations: Tailor AI governance frameworks to specific sectors, recognizing unique challenges and risks. Integrate existing legal structures and data protection laws into new AI governance models.
The future of AI governance lies in a carefully balanced combination of regulatory mechanisms. Only through this tailored, multi-layered approach can AI’s transformative potential be realized for the common good, driving inclusive growth, sustainability, and ethical progress.